Created by Brenton Crawford and Liam Webb.
The average core photo costs approximately 20 cents to collect, equating to around 4-5 cents per metre.
It contains a range of compositional and textural information that benefits both geologists and geotechnical engineers.
Why don’t we treat the collection and analysis of these data with the same rigor as we do with geochemistry or downhole geophysics?
Not just a pretty picture – imagery is data
The average state of core photography is improving, but there is still a fundamental misconception about the value and purpose of core imagery. Historically core photos have been collected as a visual record of what was drilled that was often gone back to for manual photo logging or validation of other datasets.
Even the humble core photograph is a quantitative source of data, no different to any other downhole dataset.
People routinely underestimate what is possible from their core imagery as a quantitative dataset. If you don’t get anything else from this blog, remember that!
A Learning Opportunity
Historical core photography comes in all shapes and sizes, particularly in exploration projects where the infrastructure for taking a good photograph can be difficult.
Photo quality killers
- Very high aspect angle of the photograph
- Highly variable or poor lighting
- Intense shadows
- Intense reflections
- Overcropping
Photo quality misdemeanours
- Minor reflections
- Multiple core boxes in one photograph
- Foreign objects on top of the core
- Resolution of the camera
What is possible with an old core photo?
The most common questions we get are related to what is possible and how good our images need to be:
- What kind of image quality and resolution is required?
- Will you be able to find X in our core?
Below we have created some helpful rules of thumb that address these:
- If you can do something manually and consistently with your eyes, it is likely possible to automate. For example, with consistent, well-taken photos, it is possible to map sericite, silica and other sometimes subtle alterations. In poor imagery, the variability between the imagery may be higher than the variability in the alteration.
- Geotech is easier than geology. Fractures are typically more consistent and clearer than geological features. Note that errors in depth will likely have a larger impact on geotechnical products, and if driller’s breaks aren’t marked/visible, then errors will be introduced.
- The fewer boxes in an image, the better. Preferably, we have one box per image. The more we are imaging, the fewer depth tie points we have and the fewer pixels per metre of rock there are.
- Inconsistent lighting/shadows are by far the most impactful in reducing the quality of an image and the scope of what can be automated.
Case Studies
Infilling historic geotech data using 15-year-old core photography at Carrapateena
- 140,000m of geotechnical data was generated by Datarock at the Carrapateena deposit using the 15-year-old historic core photography.
- Datarock produced a universal interpretation that unites exploration, resource definition, and production datasets.
- Significant OBK value realised from a small initial investment in good photography during exploration and resource definition phases.
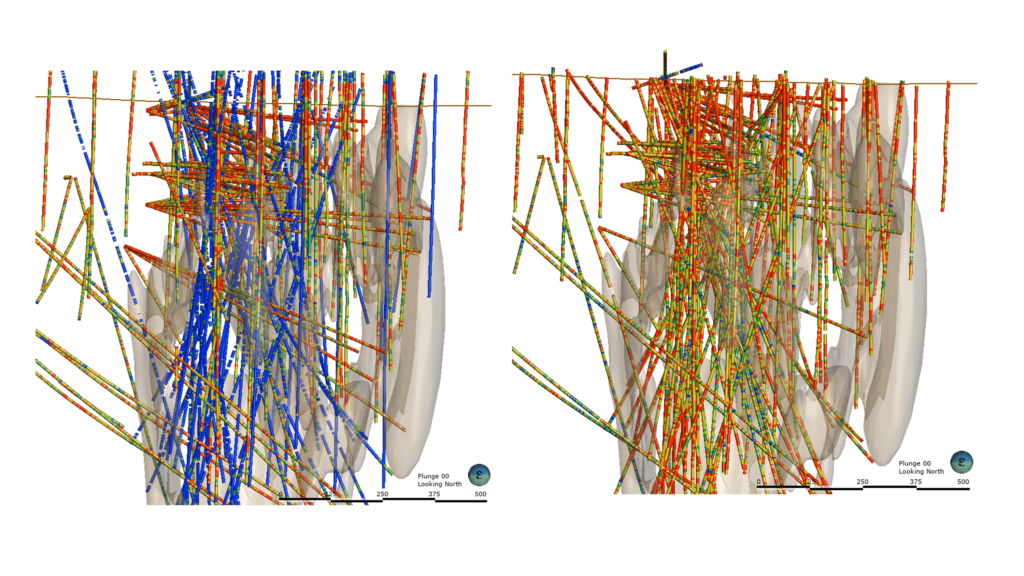
(left) Data coverage pre-Datarock analysis (blue drill strings had poor quality data); (right) data coverage post Datarock core photo analysis.
Logging vein data using core photos at Sunday Creek
- Datarock produced a quantified vein logging product on 16,000m of core photography at the Sunday Creek deposit.
- Augmented a time-consuming and subjective vein-logging dataset with low spatial coverage.
- Created a deposit-wide vein area dataset that improved OBK using their existing core photography.
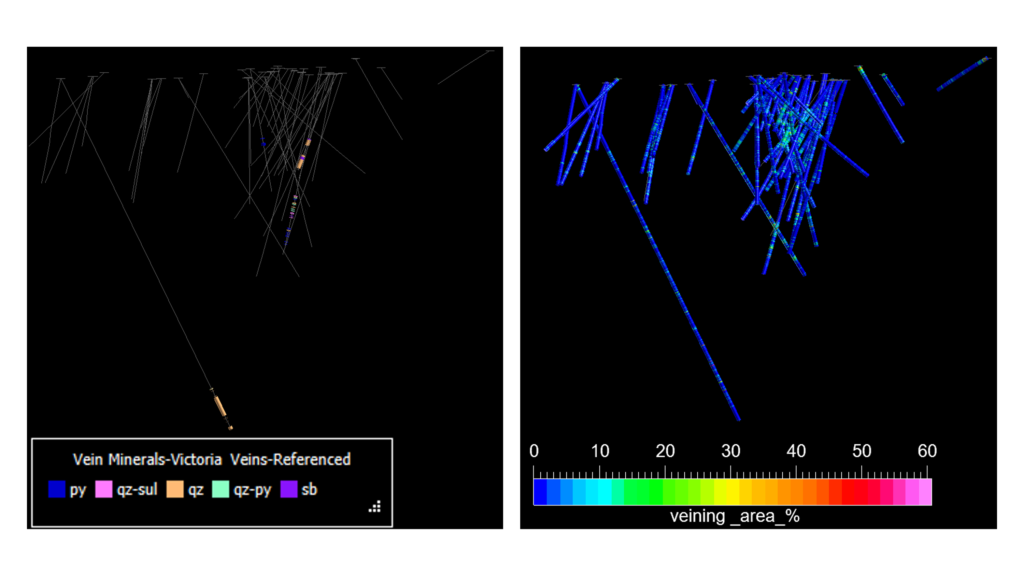
(left) Coverage of vein logging colours by dominant vein type; (right) quantitative total vein area as a percentage of the total rock area (created from the detection of 5 different vein classes).
Reach out here for more information on these and other case studies.
Why is some variation okay?
Our tendency with datasets that aren’t perfect is to try to recollect the perfect dataset, rather than trying to maximise the value of what has already been collected.
Modern machine learning algorithms are very good at understanding variation in data provided they are correctly trained. Companies routinely exclude core photography due to its perceived lack of quality without factoring in the flexibility of machine learning methods to clean the data and maximise the information able to be extracted.
A simple analogy
A machine learning model has the ability to learn that an apple is an apple, regardless of whether it’s green or red – provided you can give examples of both green and red apples to the model. It’s the same with geology – if lithologies or alterations appear differently in photos due to the camera systems or lighting, we need to capture this variability in the training data. There are limits of course, but sometimes plenty of value can be extracted before the limit is encountered.
Example: Processing a poor-quality core photo
Just because an image hasn’t been taken “perfectly” does not mean there is no analytics value – quite the opposite. Below we have a low-quality core photo taken over a decade ago that has been processed by Datarock for several geotechnical and colour variables without any model tuning or modification.
Here is the processed version of this core box where each fragment in the tray has been detected, measured and classified.
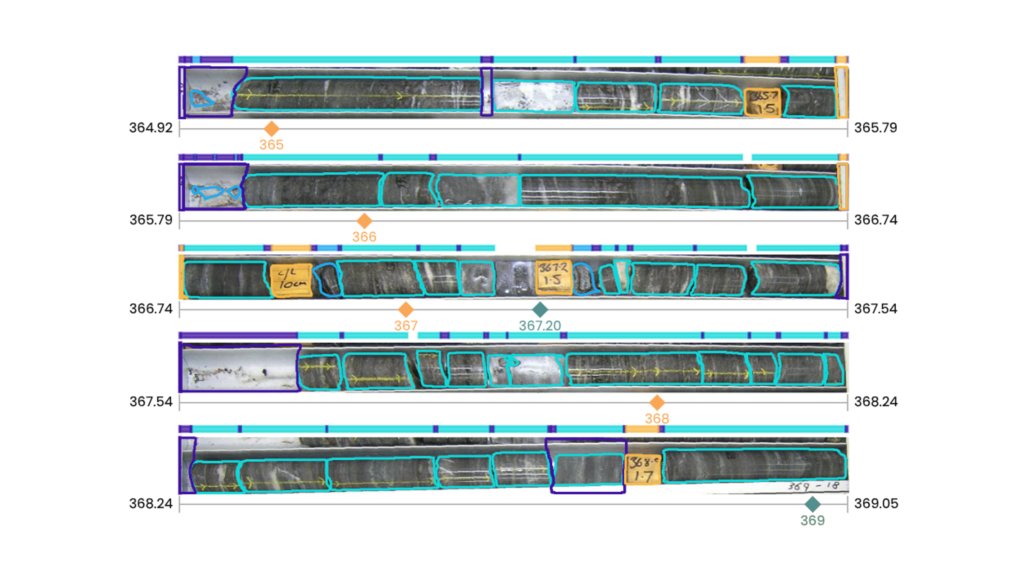
Depth registered strip imagery from the first box in the previous image. The rock has been segmented and classified with our depth registration artefacts.
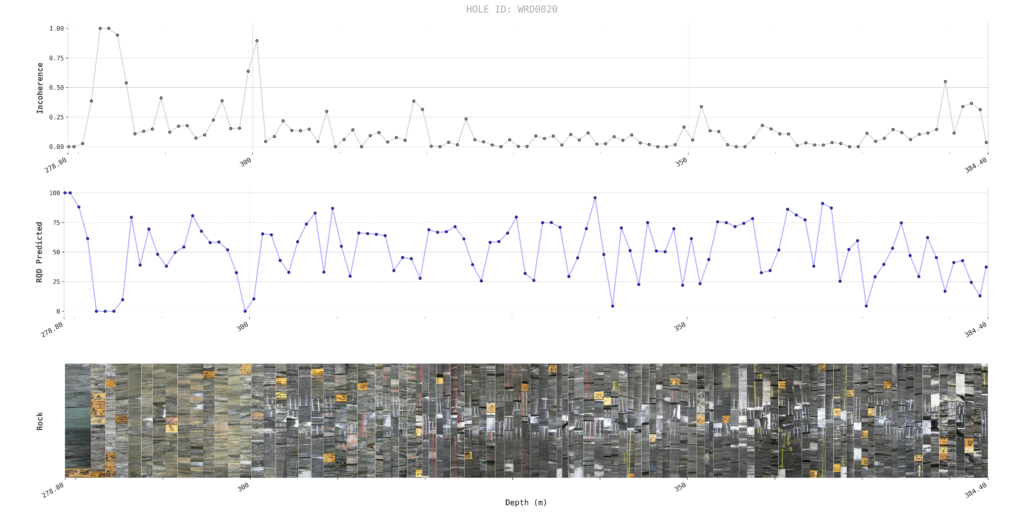
Example Datarock RQD and broken rock (incoherence) analysis across an entire drill hole of low quality imagery.
Maximising the value
Today will be the past soon, and companies are creating their next generation of historical core photography every day. If you want to maximise the value of your core photography check out our previous blog on How to take an analytics-ready core photograph. Making simple changes now will reward you in the future when the time comes to turn those unloved core photos into a valuable asset.
Why historic core photos are buried treasure… and why you need to dig them up!
- They represent one of the most long-lived and high-coverage datasets available at most mines and exploration projects
- They are cheap to collect
- They are extremely data-rich
- The visual/textural/mineralogical information they provide is not present in the most commonly relied-upon datasets (e.g. geochemistry)
- Traditional RGB core photos are as close a record as we have to what the human eye sees, allowing us to replicate traditionally visually logged datasets
At Datarock, we think core photography and the information you extract from them are Foundational. Foundational data is consistent, high resolution, auditable and – importantly here – has high spatial coverage. Because core photography is typically obtained on all drilling, it is one of the best datasets available to create foundational datasets which can underpin future decision making in the mining cycle.
At Datarock we are all about maximising value from your data using machine learning and data science.
If you want to know more about how we can help you unlock the value of your data then get in touch!