Created by Luisa Herrmann and Brenton Crawford.
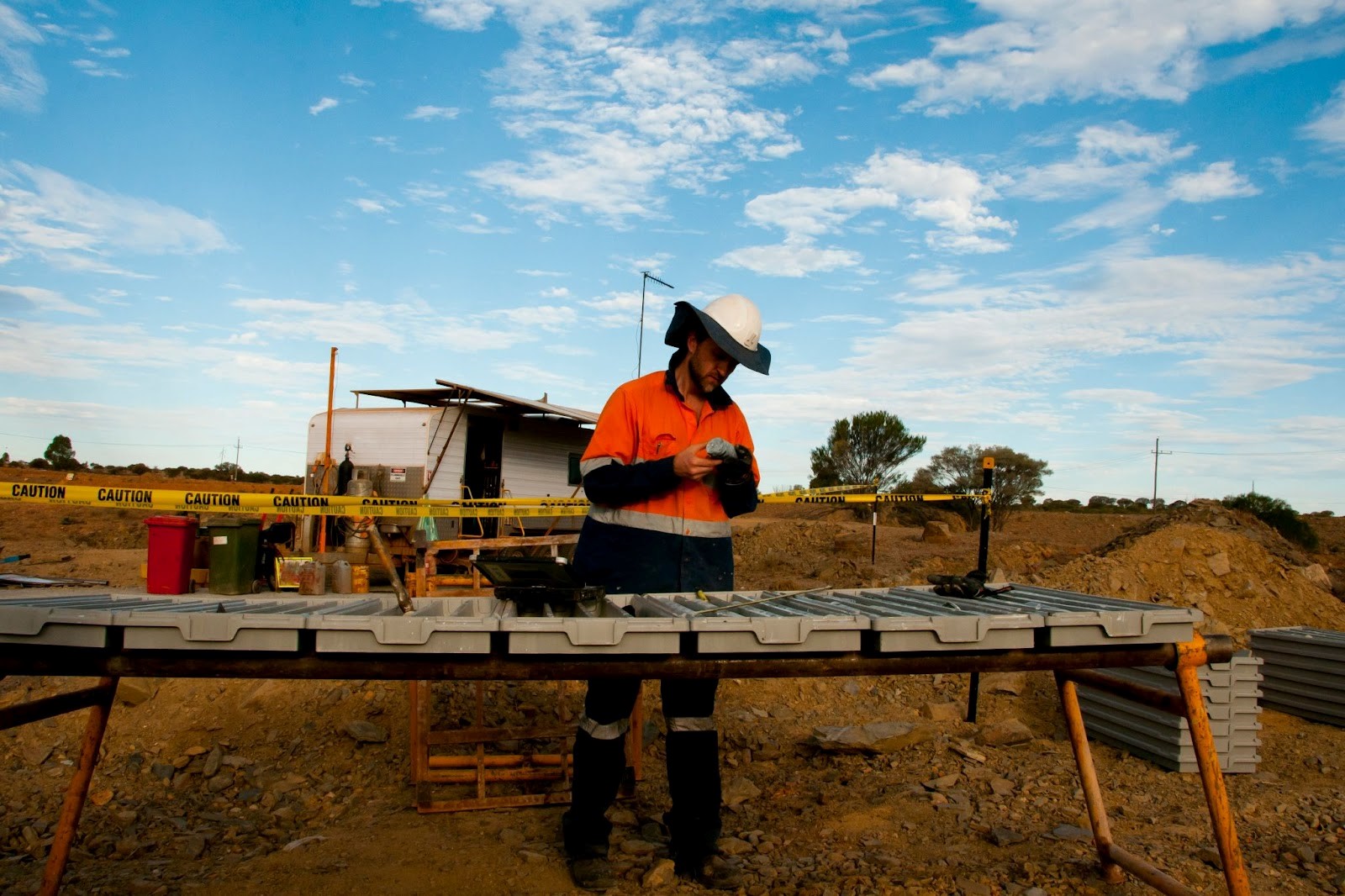
I want AI to take the dull geology jobs so that I can explore…
No!
In a previous Datarock blog post article, I wrote a response to “Will AI take geologists jobs?”, a title that was deliberately provocative to address the persistent fears of many geoscientists since the AI hype began. The blog post was one of the most commented articles we’ve written, with the most common comment being a simple “No!”. And we agree! While we work with machine learning models every day at Datarock, there’s no way we want to take any job away from an experienced geologist or geotechnical engineer. So having addressed the dramatically negative question that might first pop up when first discussing AI, it’s time to talk about the changing role of the geoscientist and how we might adapt, and lean on the positives, of this new world of augmented geoscience.
The Dull, the Dirty, the Dangerous and the Dear ($)
The AICD wrote a great article on the growing role of AI in the boardroom (https://www.aicd.com.au/innovative-technology/digital-business/artificial-intelligence/the-growing-role-of-artificial-intelligence-in-the-boardroom.html). One of the guiding principles advised to directors and senior management was to assign the 4D tasks – the dull, dirty, dangerous and dear (expensive) – to AI as much as possible. We’re seeing the pulse of this sentiment in the social landscape right now, with some humorous posts imploring AI to do the dirty jobs and leave us humans to the tasks where we excel – judgement, creativity, problem-structuring, communication, reasoning, logic and emotional intelligence.
The Dull
In the mining context we’ve already seen evidence of the dirty and dangerous being assigned to AI in the form of driverless trains and trucks controlled by remote centres of operation and automated blast machinery. In the geoscience space, we deal with many daily tasks that could be perceived as dull – not because there isn’t incredibly valuable insight to be gained from their doing – but ‘dull’ due to their repetitive nature, and need for an absolutely consistent output.
If we take vein logging as an example, a geologist might log the number and location of quartz veins in drill core from a porphyry deposit, with the aim of mapping out the big picture vein density across a deposit. Perhaps the orientation is also logged, and perhaps that tells the geoscientist something about where the deposit is placed in the alteration system, or provides an indication of its rock strength. The same logging behaviour is repeated over many hours and days, weeks and months, until each metre of core has been logged…
If we gave this repetitive task to the Datarock Platform, we could ingest many thousands of metres of core imagery, create a bespoke quartz vein segmentation model, train and test, and then run it across the entire ore deposit. Once set up, a quartz vein segmentation model could output quantitative information, such as area and count, consistently, and rapidly, all before your morning coffee gets cold. A great problem to yeet to AI! Now that the fundamental vein dataset has been created, it’s time for Human Intelligence to launch off Artificial Intelligence, and for the geoscientist to interpret the paragenesis of veins, how they relate to mineralisation or alteration or rock strength, all based on the human experience combined with logic, judgement, and perhaps a little creativity!
Machine learning as a capability booster?
Let’s say a vein dataset is modelled using AI, and the information provided across the ore deposit is entirely new to a geology team… how can a geologist review it? Is it useful as an accelerant to better understand the orebody?
An example below from a recent project where a quartz vein model was run and the output dataset imported into 3D geology software. Geological models were created based on quartz vein volume being greater than 25%, a metric that was deemed important in a porphyry – transitional to epithermal – geological setting. The results highlighted prominent stockwork corridors, and a concentration of vein density proximal to intrusive contacts. All of this was intuitive to the geoscientists, but never before did they have the quantitative dataset to visualise the veins across the orebody and gain such insight into the relationship between vein attitude and mineralisation.
(Left) Mineralization domains (transparent) relative to the newly constructed stockwork corridors based on the vein density data (opaque).
(Right) The numeric models help contour the data and show the localised areas of concentrated vein density, many of which appear at intrusive contacts.
Human validation of Machine Learning and Machine Learning to review human decision-making
Many of the readers of this blog post will have spent time logging RQD in a core shed, and know that Datarock can automate the logging of RQD through our Platform. It’s astoundingly quick too – a recent project generated 30,000m of RQD in 15 mins! So what does the geoscientist do when there is both a machine learning model and a human derived model of the same dataset? It’s a wonderful, and rare, opportunity to cross check datasets, and we encourage it! Where lithology logging can be refined by assay results, RQD doesn’t typically have an external validation dataset available. So here is AI, boosting the capability of the geotechnical engineer, adding a layer of information to validate findings and show discrepancies, ultimately to create better input into geotechnical models.
An example of Ground Truth (customer) RQD vs Predicted RQD generated from the Datarock Platform. Where the two datasets divulge is an opportunity for validation of the human derived model.
The key is harmonisation
As we delve deeper into the use of AI in geoscience, the conversations we are having with customers are steadily changing, from fearful, to recognising its use as an accelerator of knowledge. Datarock’s mission is to be part of that change, supporting the creation of data-driven cultures in which geoscientists have the willingness to use their own data, to drive their own decisions, in collaboration with their own gut feel.
Ultimately, we, as geoscientists, need to use AI to our advantage. To create new insights that support our intuition, and use it to validate existing human-generated datasets so that we might gain trust that AI generated datasets can be used in the future.
Far from AI taking our jobs, we believe that developing skills and knowledge about AI – learning how to interpret its recommendations, and integrating it into our workflows – is the key to keeping our jobs.